Challenges and Solutions in Demand and Forecasting Analysis
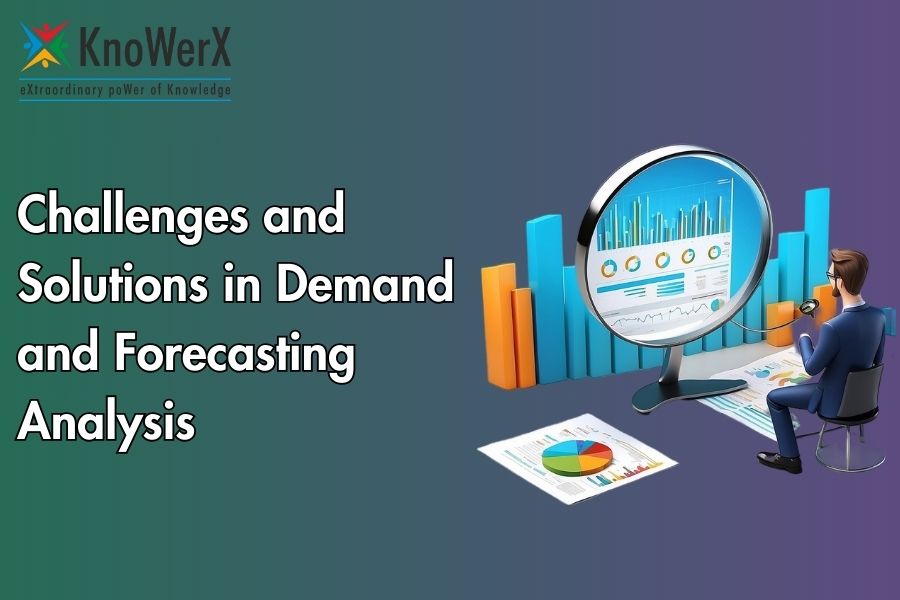
Addressing Key Challenges and Solutions in Demand and Forecasting Analysis Demand and forecasting analysis plays a crucial role in modern supply chain management, helping businesses anticipate customer needs, optimize inventory levels, and enhance overall operational efficiency. Accurate demand forecasts are essential for making informed decisions that directly impact profitability and customer satisfaction. However, the process of demand and forecasting analysis is fraught with challenges, ranging from data quality issues to adapting to market volatility. This article delves into the common challenges associated with demand and forecasting analysis and explores effective solutions, future trends, and the importance of education and training in this critical area. Understanding Demand and Forecasting Analysis Demand and forecasting analysis involves predicting future customer demand for products or services based on historical data, market trends, and other relevant factors. This analysis is vital for businesses to align their production, inventory, and supply chain strategies with anticipated demand. Various methods, such as qualitative techniques (e.g., expert opinion) and quantitative techniques (e.g., time series analysis), are used in demand forecasting to estimate future demand accurately. The significance of demand and forecasting analysis cannot be overstated, as it directly influences business decision-making. Effective demand forecasting enables companies to avoid stockouts, minimize excess inventory, reduce costs, and improve customer satisfaction. However, achieving accuracy in demand forecasting is a complex task that requires addressing several challenges inherent in the process. Challenges in Demand and Forecasting Analysis Data Quality Issues: Data quality is a fundamental aspect of demand and forecasting analysis. Inaccurate or incomplete data can lead to inaccurate forecasts, resulting in insignificant decisions. Businesses often struggle with collecting reliable historical data, especially in industries with rapidly changing consumer preferences or where data is fragmented across different sources. Poor data quality can distort demand predictions, leading to overproduction or stockouts, both of which are disastrous to business operations. Volatility and Market Changes: The dynamic nature of markets presents a significant challenge in demand and forecasting analysis. Sudden shifts in market conditions, economic fluctuations, and unpredictable demand patterns can render traditional forecasting models ineffective. For example, unexpected events like a global pandemic can drastically alter consumer behaviour, making it difficult for businesses to forecast demand accurately. Adapting to such volatility requires a flexible approach to demand forecasting that can quickly respond to changes in real-time. Complexity in Demand Patterns: Demand patterns are often influenced by various factors, including seasonality, trends, and cyclical behaviour. Identifying and interpreting these patterns is crucial for accurate demand forecasting. However, the complexity of demand patterns can pose challenges, especially in industries where multiple factors interact simultaneously. Businesses must navigate the intricacies of seasonal variations, long-term trends, and short-term fluctuations to generate reliable forecasts. Technological and Analytical Challenges: The integration of advanced forecasting tools and technologies is essential for enhancing the accuracy of demand forecasts. However, many businesses face challenges in adopting these technologies due to limitations in analytical capabilities and resources. Implementing sophisticated forecasting models, such as those based on machine learning and AI, requires a deep understanding of the underlying algorithms and access to vast amounts of data. Additionally, small and medium-sized enterprises may struggle with the costs and expertise required to deploy advanced forecasting tools effectively. Solutions to Overcome Challenges Improving Data Quality: To address data quality issues in demand and forecasting analysis, businesses should prioritize the collection of accurate and comprehensive data. Implementing robust data cleansing and validation processes can help eliminate errors and inconsistencies in the data. Additionally, businesses can invest in data management systems that consolidate information from various sources, ensuring that forecasting models are built on reliable data. By enhancing data quality, companies can significantly improve the accuracy of their demand forecasts. Adapting to Market Volatility: In an environment characterized by market volatility, businesses must adopt flexible and adaptive forecasting models. Scenario planning, which involves creating multiple demand forecasts based on different potential market conditions, can help businesses prepare for uncertainty. Real-time data integration is also critical for responding to sudden market changes. By continuously monitoring market trends and adjusting forecasts accordingly, businesses can stay agile and better align their operations with actual demand. Enhancing Analytical Tools: The use of advanced analytical methods, such as machine learning and AI, can greatly improve the accuracy of demand forecasts. These technologies can analyse vast datasets, identify patterns, and generate more precise predictions. Businesses should consider investing in big data and predictive analytics tools that allow for deeper insights into customer behaviour and demand trends. Additionally, cloud-based forecasting solutions can provide scalability and flexibility, enabling businesses to leverage cutting-edge technologies without the need for extensive infrastructure. Collaboration and Cross-functional Teams: Effective demand and forecasting analysis often require collaboration across different departments within an organization. Cross-functional teams, composed of members from sales, marketing, finance, and supply chain, can provide diverse perspectives that enhance the accuracy of demand forecasts. By involving various departments in the forecasting process, businesses can ensure that all relevant factors are considered, leading to more comprehensive and reliable forecasts. Future Trends in Demand and Forecasting Analysis Integration of AI and Machine Learning: As AI and machine learning technologies continue to advance, their integration into demand and forecasting analysis is expected to become more widespread. These technologies can automate the forecasting process, enabling businesses to generate accurate predictions with minimal human intervention. Future developments may include self-learning forecasting systems that continuously improve their accuracy by analysing new data and adapting to changing market conditions. Emphasis on Real-time Data: The importance of real-time data in demand and forecasting analysis is growing, driven by the increasing availability of IoT and smart technologies. Real-time data allows businesses to monitor demand patterns as they occur, enabling them to make swift adjustments to their forecasts. In the future, real-time data integration will likely become a standard practice, providing businesses with the ability to respond to market changes in near real-time. Sustainability and Green Supply Chain Forecasting: As sustainability becomes a priority for businesses, demand and forecasting analysis will need to incorporate considerations related to environmental impact. Forecasting for sustainable